Haw-Shiuan Chang
張浩軒
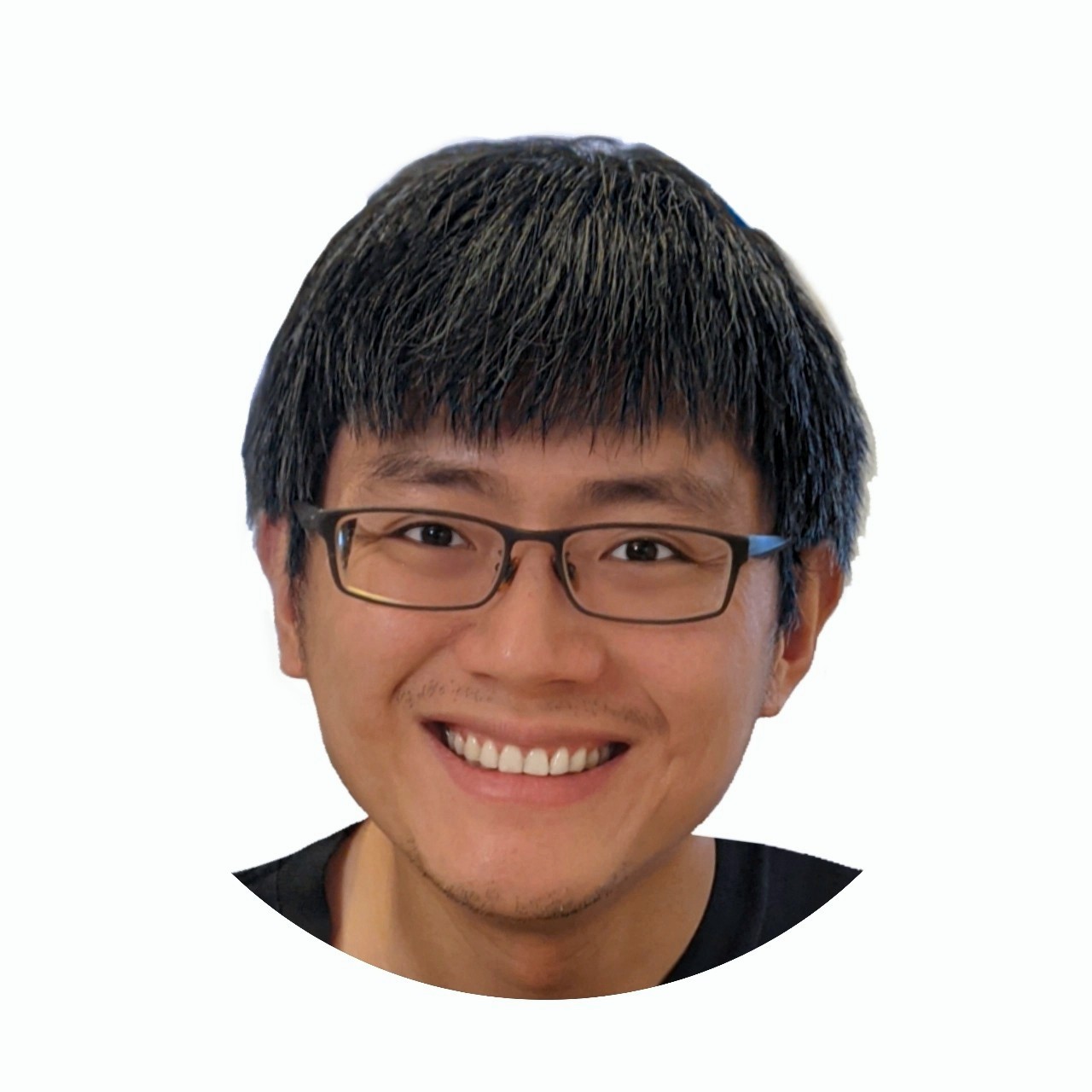
I am a postdoc in the faculty job market now. My current position is a postdoctoral research associate at UMass Amherst Center for Intelligent Information Retrieval (CIIR), advised by Professor Hamed Zamani. I am broadly interested in developing intelligent systems that could assist humans in their creative process. Currently, I focus on fundamentally narrowing the gap between large language models (LLMs) and human intelligence without relying on scaling laws such as increasing model size or training data. Toward that end, I am particularly interested in
(1) enhancing the generation factuality, diversity, and novelty of LLMs by encouraging the usage of more causal inferences and fewer guesses,
(2) discovering LLMs’ limitations in their current architectures, decoding algorithms, evaluation, and training data, and
(3) overcoming the limitations through the techniques inspired by human behavior, machine learning (ML), and information retrieval (IR).
Previously, I was a postdoctoral scientist at Amazon AGI Foundations and worked with Professor Violet Peng, Professor Mohit Bansal, and Dr. Tagyoung Chung. I got my PhD at the University of Massachusetts Amherst, advised by Professor Andrew McCallum. Prior to PhD, I worked with Professor Yu-Chiang Frank Wang and Dr. Kuan-Ta Chen at Academia Sinica, Taiwan. I received my BS in the EECS Undergraduate Honors Program from National Yang Ming Chiao Tung University (NYCU), Taiwan.