Projects
Extrapolating the Distributions of an Infinitely-Large Language Model
Why Does Contrastive Decoding Work Well and How we could make it Better?
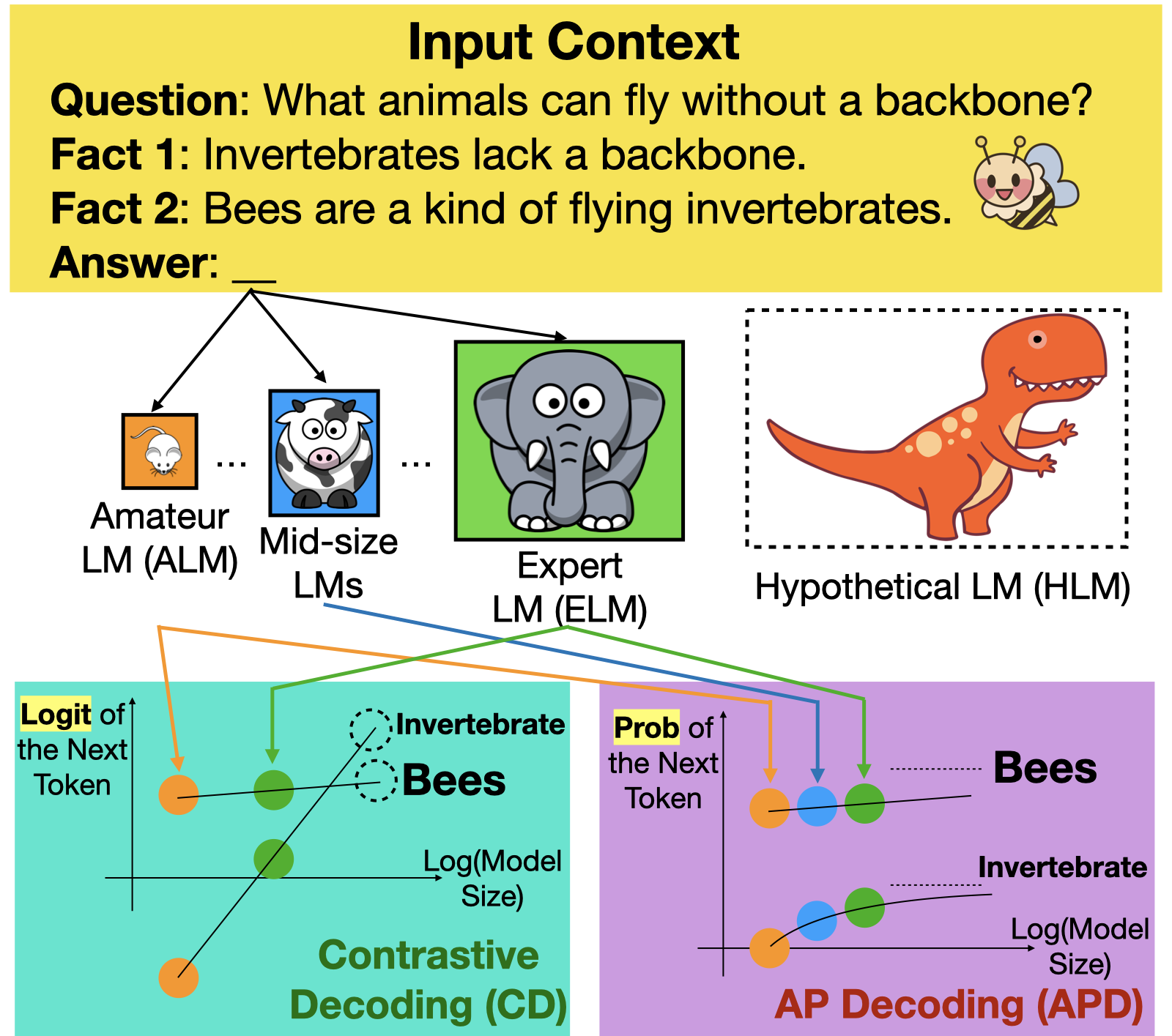
Be Careful when LLM is more Uncertain than it Should Be.
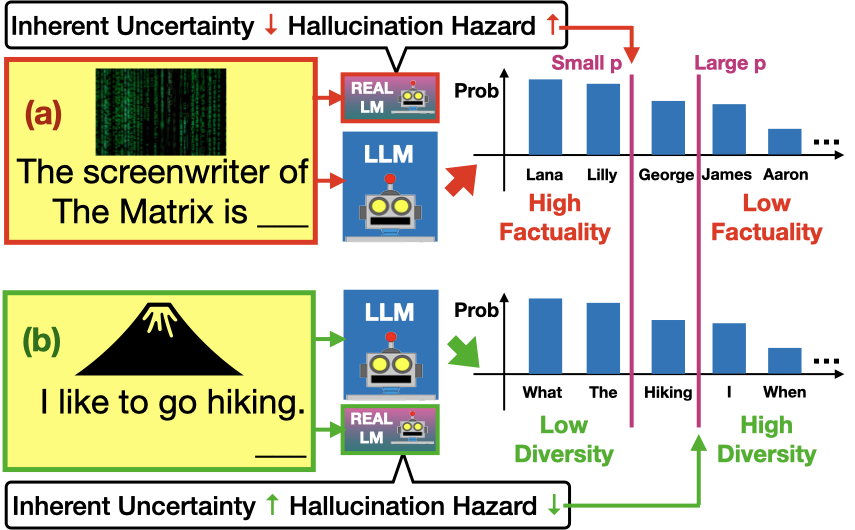
We propose REAL (Residual Entropy from Asymptotic Line) sampling, a decoding method that achieves improved factuality and diversity over nucleus sampling by predicting an adaptive threshold of p. Specifically, REAL sampling predicts the step-wise likelihood of an LLM to hallucinate, and lowers the p threshold when an LLM is likely to hallucinate. Otherwise, REAL sampling increases the p threshold to boost the diversity.
To predict the step-wise hallucination likelihood without supervision, we construct a Token-level Hallucination Forecasting (THF) model, which predicts the asymptotic entropy (i.e., inherent uncertainty) of the next token by extrapolating the next-token entropies of an infinitely large language model from a series of LLMs with different sizes. If a LLM's entropy is higher than the asymptotic entropy (i.e., the LLM is more uncertain than it should be), the THF model predicts a high hallucination hazard, which leads to a lower p threshold in REAL sampling.
After combined with contrastive decoding, REAL sampling outperforms 9 sampling methods, and generates texts that are more factual than the greedy sampling and more diverse than the nucleus sampling with p=0.5 in the FactualityPrompts benchmark. (Paper, Code)
Multi-facet Embeddings for Language Modeling
In this project, we study the theoretical limitations of the single context embedding in LMs and how the theoretical analyses suggest new alternative softmax layers that encode a context as multiple embeddings. The proposed alternatives achieve better perplexity than the mixture of softmax (MoS), especially given an ambiguous context, without adding significant computational cost to LMs. Our approaches also let GPT-2 learn to properly copy the entities from the context, which increases the coherence of the generated text without requiring any labels. In addition to predicting the next word, we also use multiple CLS embeddings to improve state-of-the-art pretraining methods for BERT on natural language understanding (NLU) benchmarks without introducing significant extra parameters or computations, especially when the training datasets are small. Furthermore, we show that our multi-facet embeddings improve the sequential recommendation, scientific paper embeddings, distantly supervised relation extraction, and cold-start citation recommendation. Finally, we use the multiple vector embeddings to predict the future topics of a context, and build on the basis, we propose a novel interactive language generation framework. (PhD Thesis, Slides)
Simply Replacing the Ouput Softmax Layer Improves Neural Sequential Recommenders by around 20% in 12 Widely-Used Datasets!
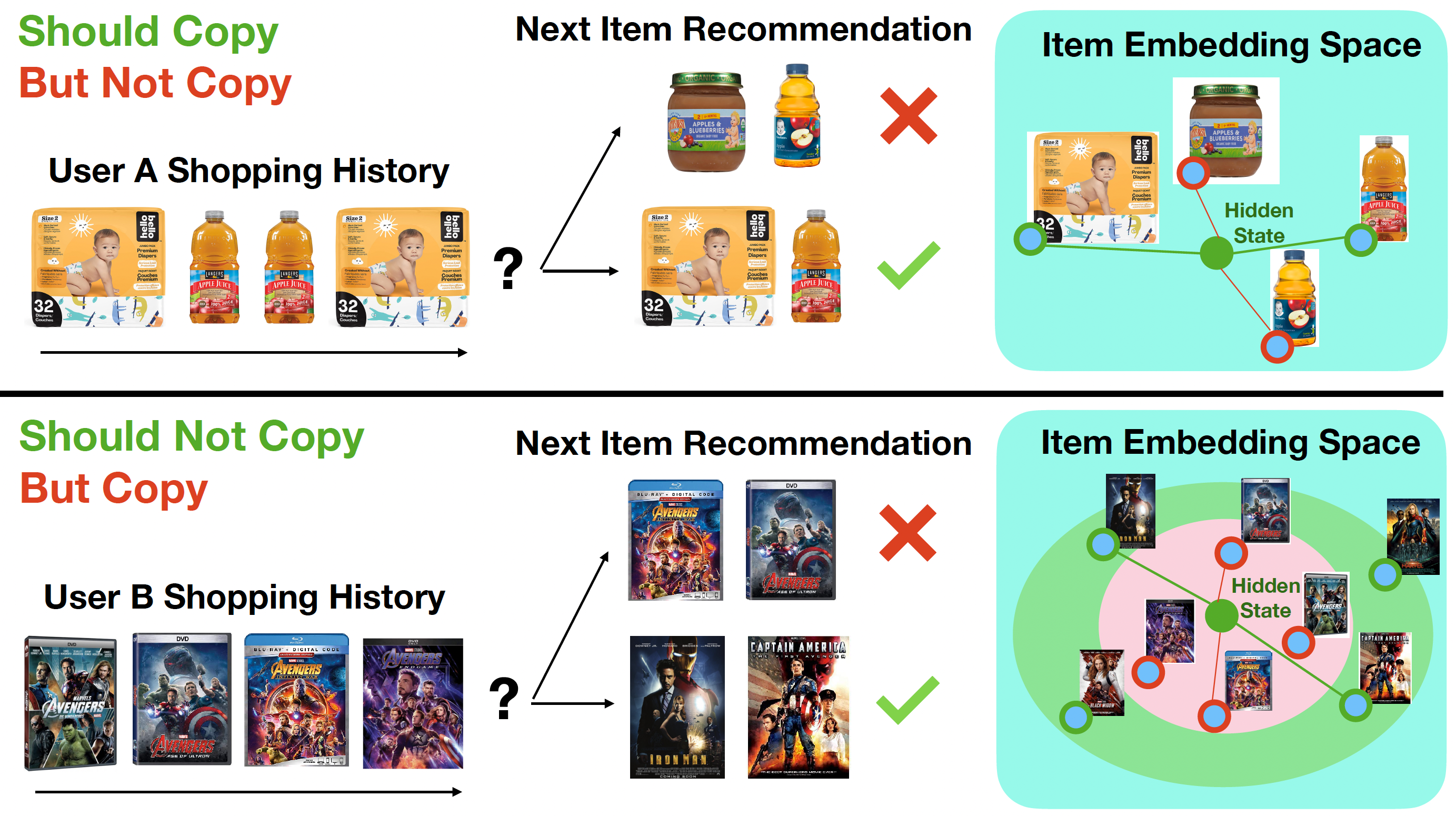
The similarity structure of the global item embeddings in the softmax layer sometimes forces the single hidden state embedding to be close to new items when copying is a better choice, while sometimes forcing the hidden state to be close to the items from the input inappropriately. To alleviate the problem, we adapt the recently-proposed softmax alternatives such as softmax-CPR to sequential recommendation tasks and demonstrate that the new softmax architectures unleash the capability of the neural encoder on learning when to copy and when to exclude the items from the input sequence. By only making some simple modifications on the output softmax layer for SASRec and GRU4Rec, softmax-CPR achieves consistent improvement in 12 datasets. With almost the same model size, our best method not only improves the average NDCG@10 of GRU4Rec in 5 datasets with duplicated items by 10% (4%-17% individually) but also improves 7 datasets without duplicated items by 24% (8%-39%)! (Paper, Code)
Why and When Pointer Networks Improve LMs and How to Do Even Better (aka It does not Make Sense to use Attention in All Transformer Layers Except the Last Softmax Layer!)
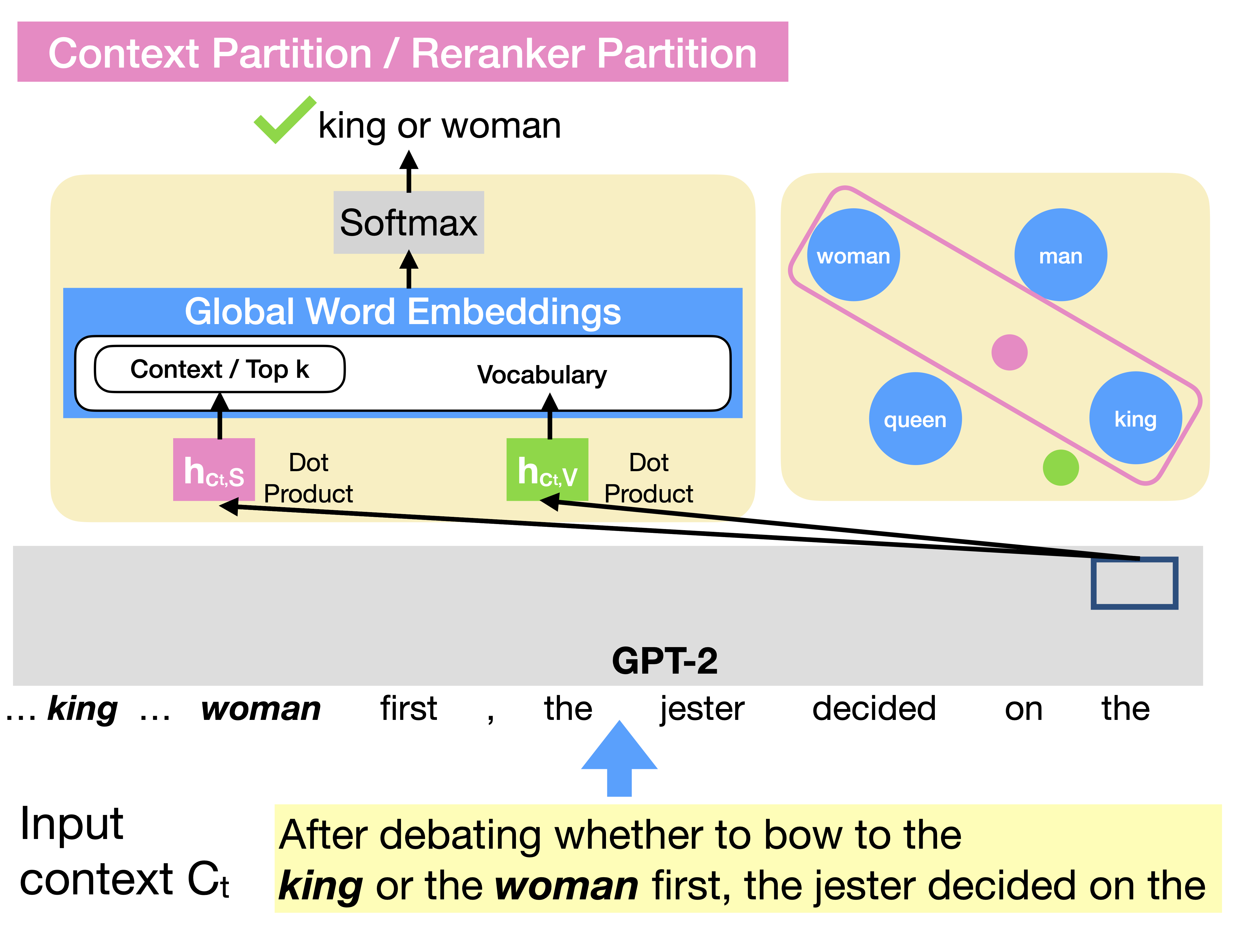
The softmax bottleneck (Chang and McCallum (2022)) sometimes prevents the language models from predicting the desired distribution and the pointer networks can be used to break the bottleneck efficiently. Based on the finding, we propose the context/encoder partition by simplifying the pointer networks and the reranker partition to accelerate the word-by-word rerankers. By combining these softmax alternatives, softmax-CPR is significantly better and more efficient than mixture of softmax (MoS) in GPT-2, a state-of-the-art softmax alternative. In summarization experiments, without significantly decreasing its training/testing speed, softmax-CEPR based on T5-Small improves factCC score by 2 points in CNN/DM and XSUM dataset, and improves MAUVE scores by around 30% in BookSum paragraph-level dataset. (Paper, Code)
Ensembling BERT almost without Additional Cost!
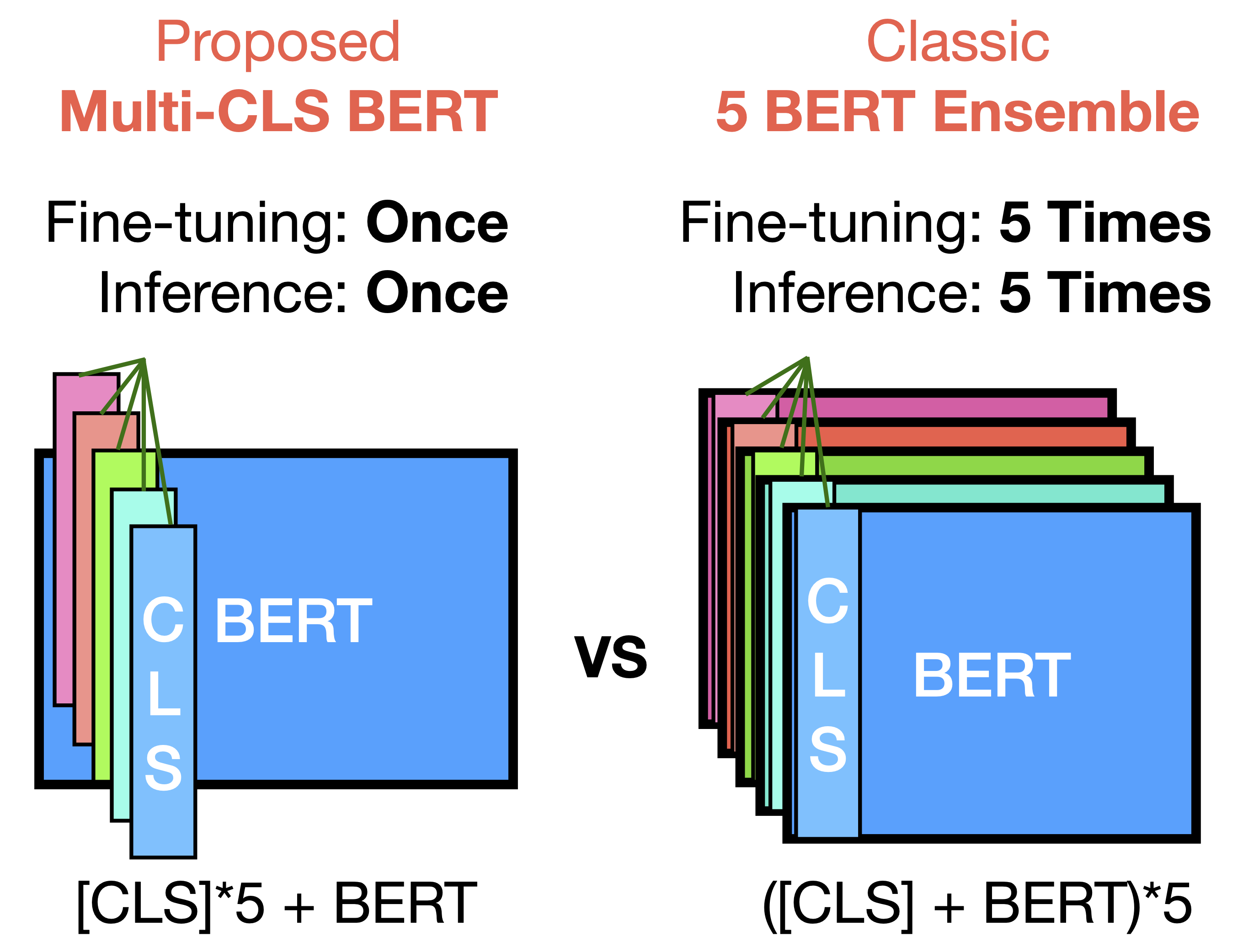
We propose Multi-CLS BERT, a novel ensembling method for CLS-based prediction tasks that is almost as efficient as a single BERT model. Multi-CLS BERT uses multiple CLS tokens with a parameterization and objective that encourages their diversity. Thus instead of fine-tuning each BERT model in an ensemble (and running them all at test time), we need only fine-tune our single Multi-CLS BERT model (and run the one model at test time, ensembling just the multiple final CLS embeddings). To test its effectiveness, we build Multi-CLS BERT on top of a state-of-the-art pretraining method for BERT (Aroca-Ouellette and Rudzicz, 2020). In experiments on GLUE and SuperGLUE we show that our Multi-CLS BERT reliably improves both overall accuracy and confidence estimation. When only 100 training samples are available in GLUE, the Multi-CLS BERT_Base model can even outperform the corresponding BERT_Large model. We analyze the behavior of our Multi-CLS BERT, showing that it has many of the same characteristics and behavior as a typical BERT 5-way ensemble, but with nearly 4-times less computation and memory. (Paper, Code) We also show that Multi-CLS BERT significantly improves multi-domain scientific paper encoders. (Paper)
Why Multiple Embeddings are Better in LM's Output Softmax Layer
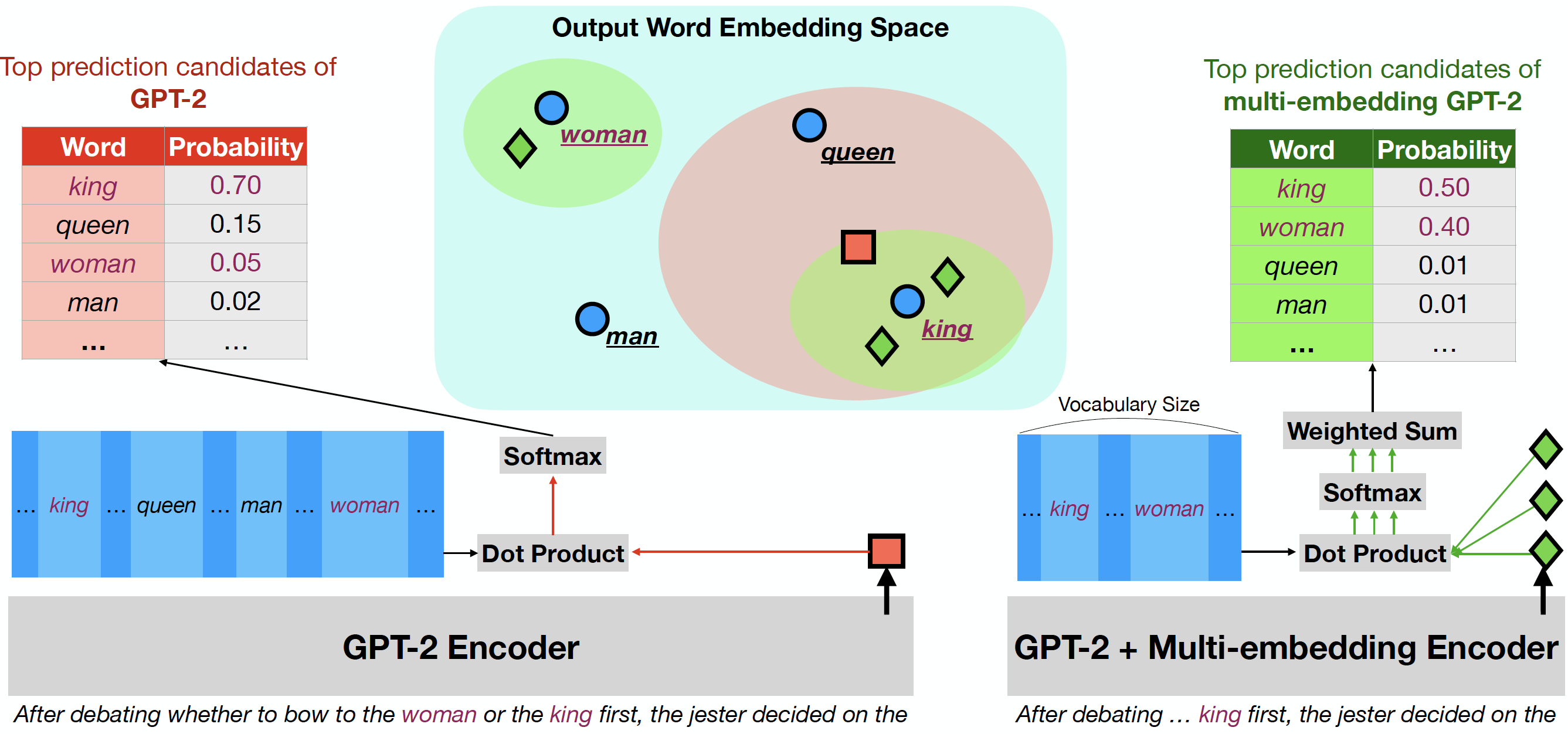
We theoretically show that this single hidden state cannot produce all probability distributions regardless of the language model (LM) size or training data size because the single hidden state embedding cannot be close to the embeddings of all the possible next words simultaneously when there are other interfering word embeddings between them. Our work not only deepens our understanding of softmax bottleneck and mixture of softmax (MoS) but also inspires us to propose multi-facet softmax (MFS) to address the limitations of MoS (Paper, Code, Talk, Slides, Poster).
Multi-facet Embeddings for Distantly Supervised Relation Extraction
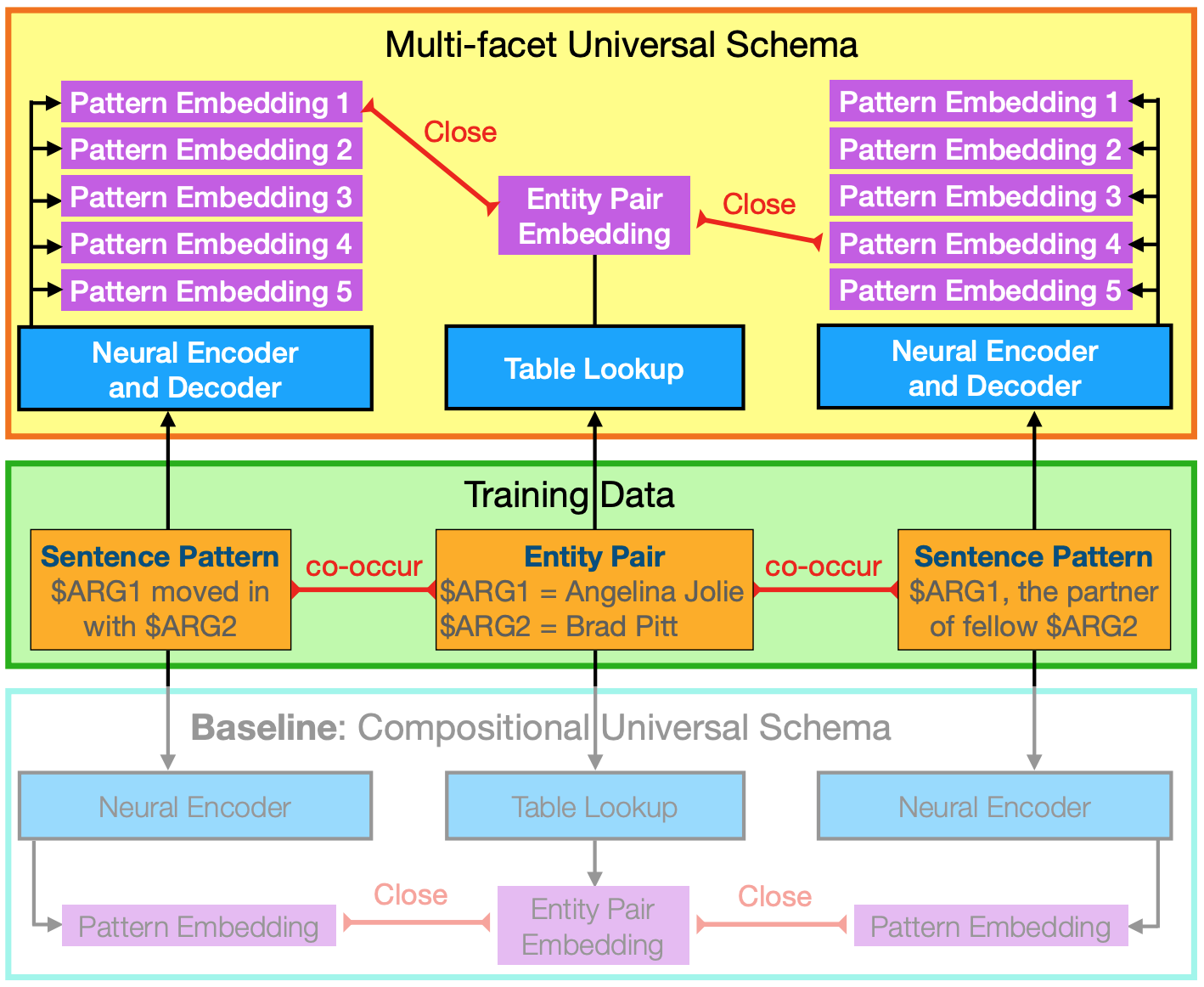
We propose multi-facet universal schema that uses a neural model to represent each sentence pattern as multiple facet embeddings and encourage one of these facet embeddings to be close to that of another sentence pattern if they cooccur with the same entity pair. In our experiments, we demonstrate that multi-facet embeddings significantly outperform their single facet embedding counterpart, compositional universal schema (Verga et al., 2016), in distantly supervised relation extraction tasks. Moreover, we can also use multiple embeddings to detect the entailment relation between two sentence patterns when no manual label is available (Paper, Code, Talk, Slides, Poster).
Predicting Cluster Centers for Sentence Representation
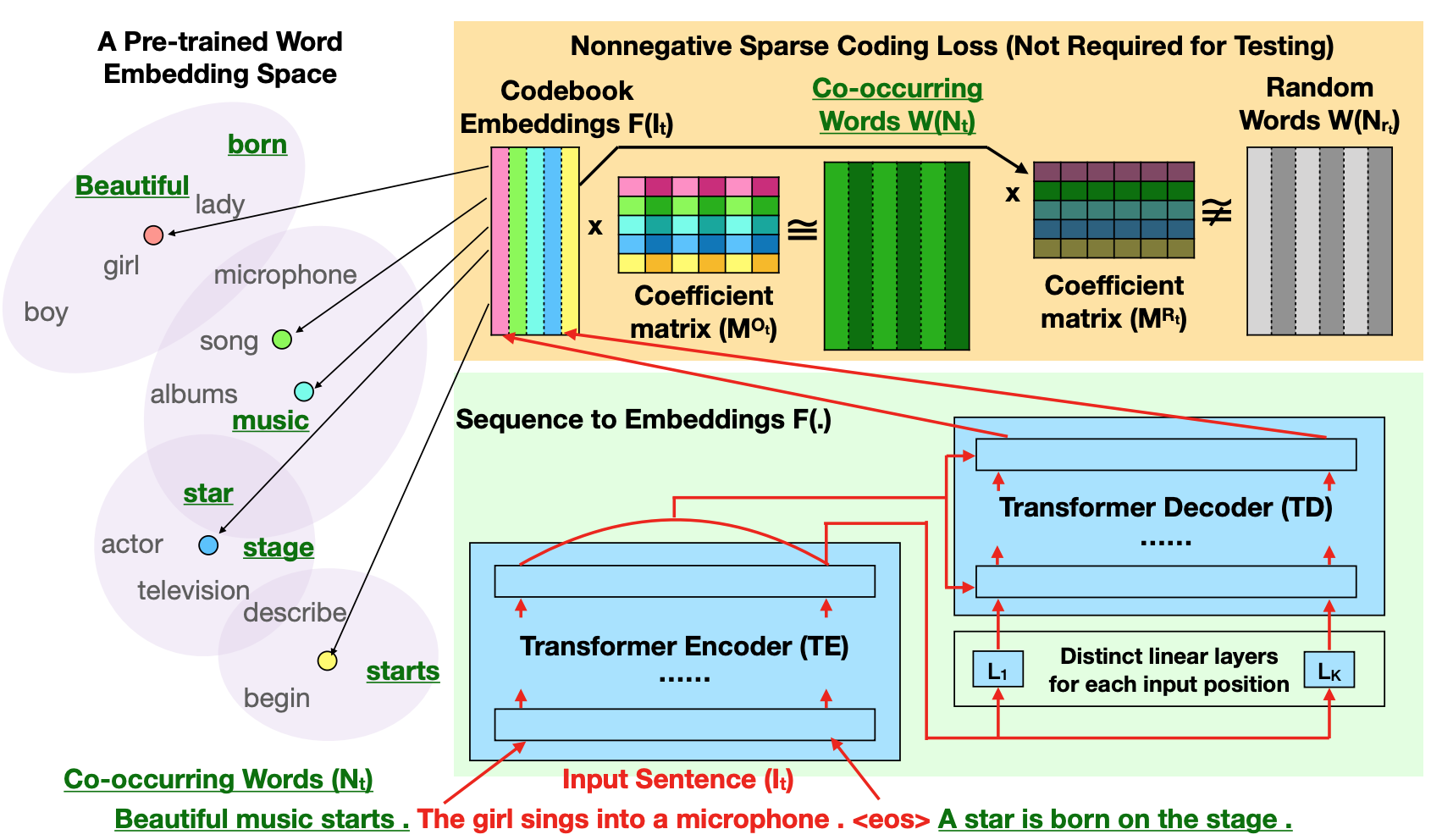
We propose a novel embedding method for a text sequence (e.g., a sentence) where each sequence is represented by a distinct set of multi-mode codebook embeddings to capture different semantic facets of its meaning. The codebook embeddings can be viewed as the cluster centers which summarize the distribution of possibly co-occurring words in a pre-trained word embedding space. Our experiments show that the per-sentence codebook embeddings significantly improve the performances in unsupervised sentence similarity and extractive summarization benchmarks (Paper, Slides, Poster).
Creative Generation of Language Models
Automatically Measuring the Creativity of Large Language Models
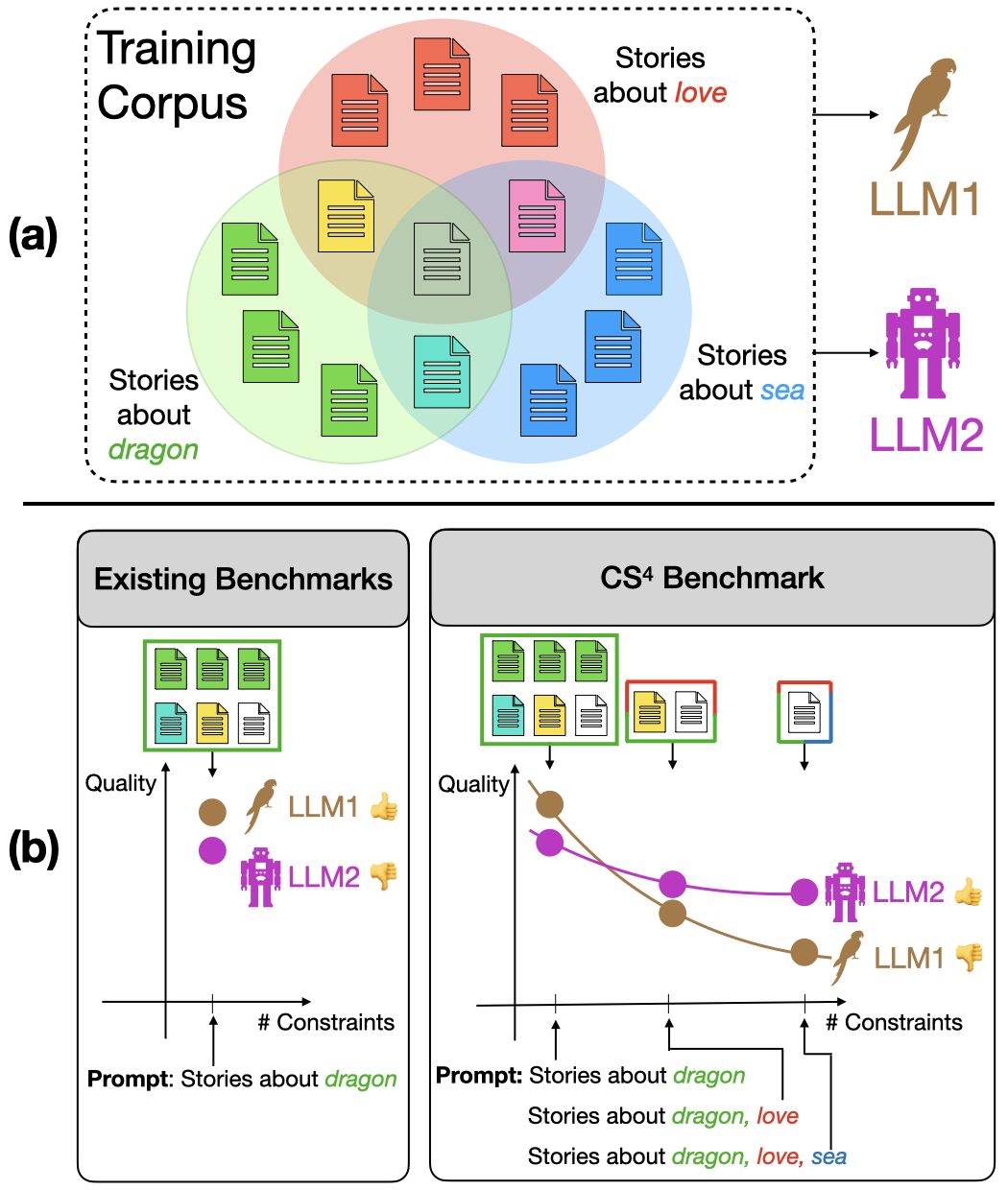
Evaluating the creativity of large language models (LLMs) in story writing is difficult because LLM-generated stories could seemingly look creative but be very similar to some existing stories in their huge and proprietary training corpus. To overcome this challenge, we introduce a novel benchmark dataset, CS4, with varying levels of prompt specificity. By increasing the number of requirements/constraints in the prompt, we can increase the prompt specificity and hinder LLMs from retelling high-quality narratives in their training data. Consequently, CS4 empowers us to indirectly measure the LLMs' creativity without human annotations.
Our experiments on LLaMA, Gemma, and Mistral not only highlight the creativity challenges LLMs face when dealing with highly specific prompts but also reveal that different LLMs perform very differently under different numbers of constraints and achieve different balances between the model's instruction-following ability and narrative coherence. Additionally, our experiments on OLMo suggest that Learning from Human Feedback (LHF) can help LLMs select better stories from their training data but has limited influence in boosting LLMs' ability to produce creative stories that are unseen in the training corpora. (Paper, Poster).
Coarse-to-Fine Story Generation by Constructing Entailment Hierarchy
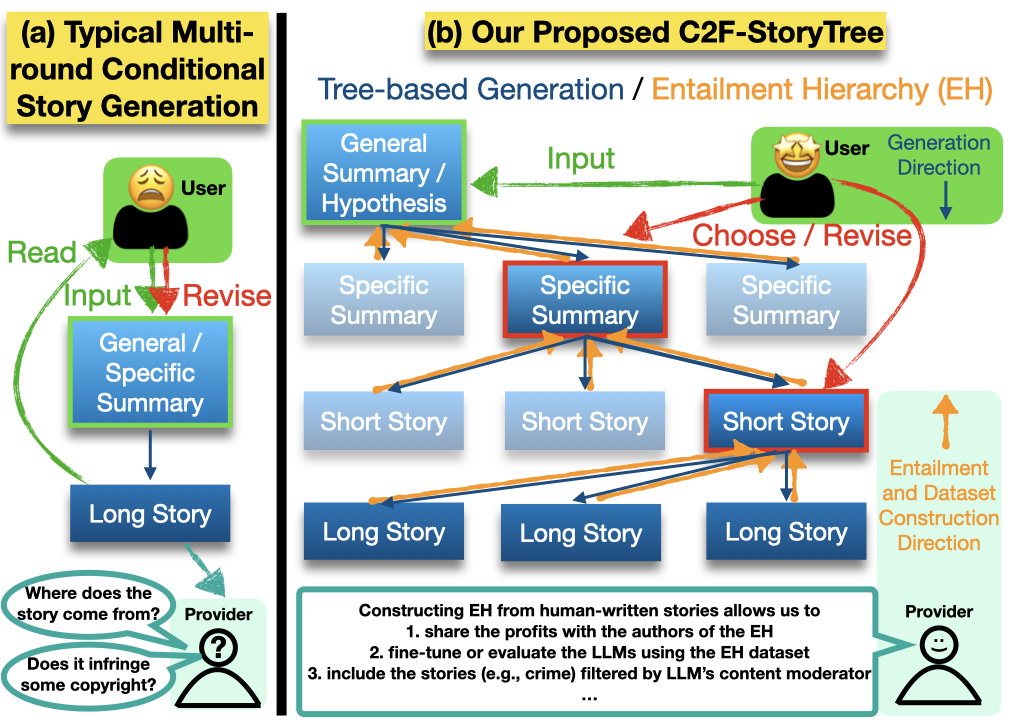
Predicting the Future Topics for Interactive Language Generation
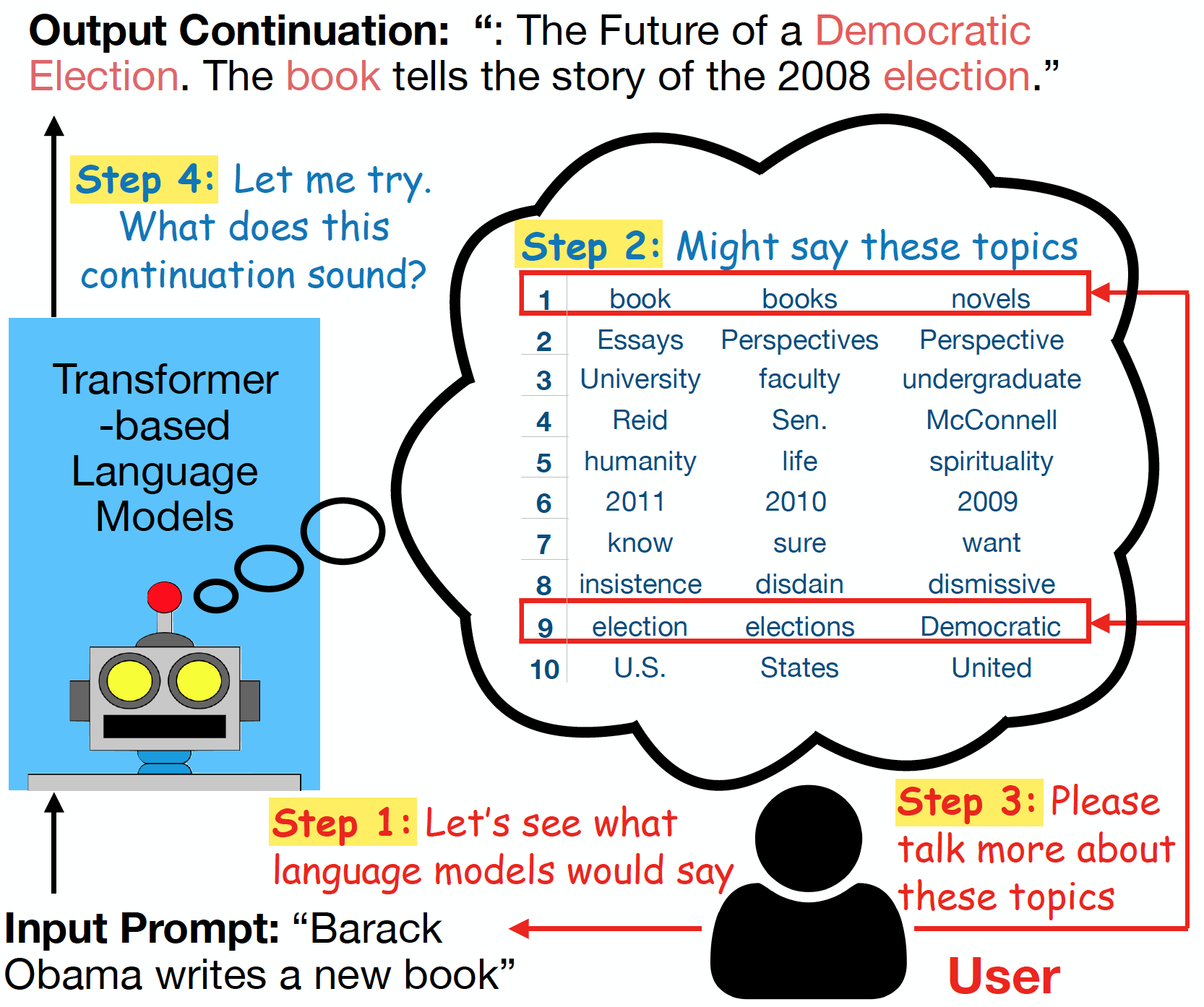
We design a framework that displays multiple candidate upcoming topics, of which a user can select a subset to guide the generation. Our framework consists of two components: (1) a method that produces a set of candidate topics by predicting the centers of word clusters in the possible continuations, and (2) a text generation model whose output adheres to the chosen topics. The training of both components is self-supervised, using only unlabeled text. Our experiments demonstrate that our topic options are better than those of standard clustering approaches, and our framework often generates fluent sentences related to the chosen topics, as judged by automated metrics and crowdsourced workers (Paper, Code, Talk, Slides, Poster).
Active Learning and Crowdsourcing
Overcoming Practical Issues of Deep Active Learning
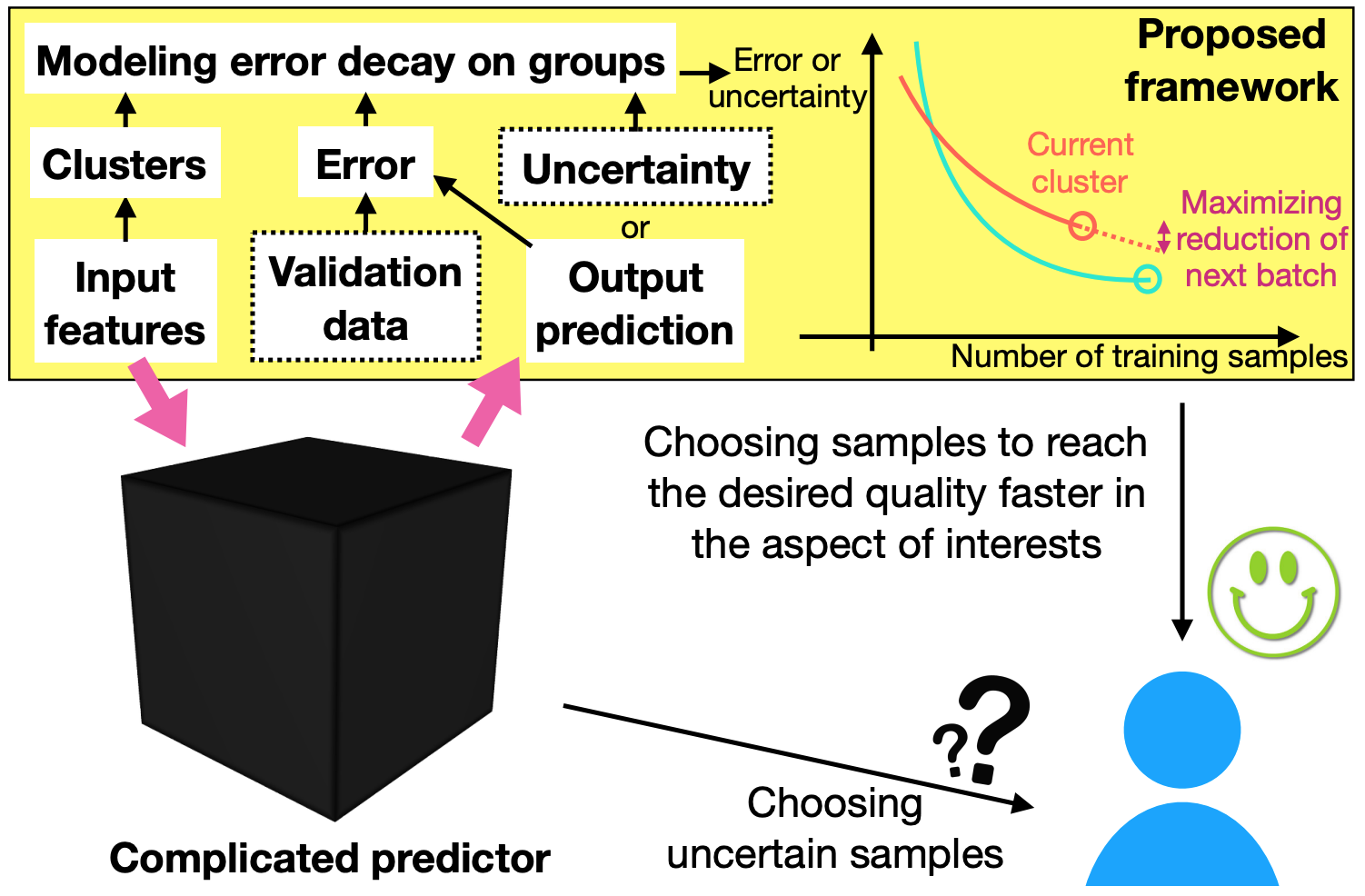
Existing deep active learning algorithms achieve impressive sampling efficiency on natural language processing tasks. However, they exhibit several weaknesses in practice, including (a) inability to use uncertainty sampling with black-box models, (b) lack of robustness to noise in labeling, (c) lack of transparency. In response, we propose a transparent batch active sampling framework by estimating the error decay curves of multiple feature-defined subsets of the data. We perform extensive experiments on four named entity recognition (NER) tasks and results show that our methods greatly alleviate these limitations without sacrificing too much sampling efficiency (Paper, Slides, Talk).
Active Sampling for Estimating Quality of Experience (QoE) Model
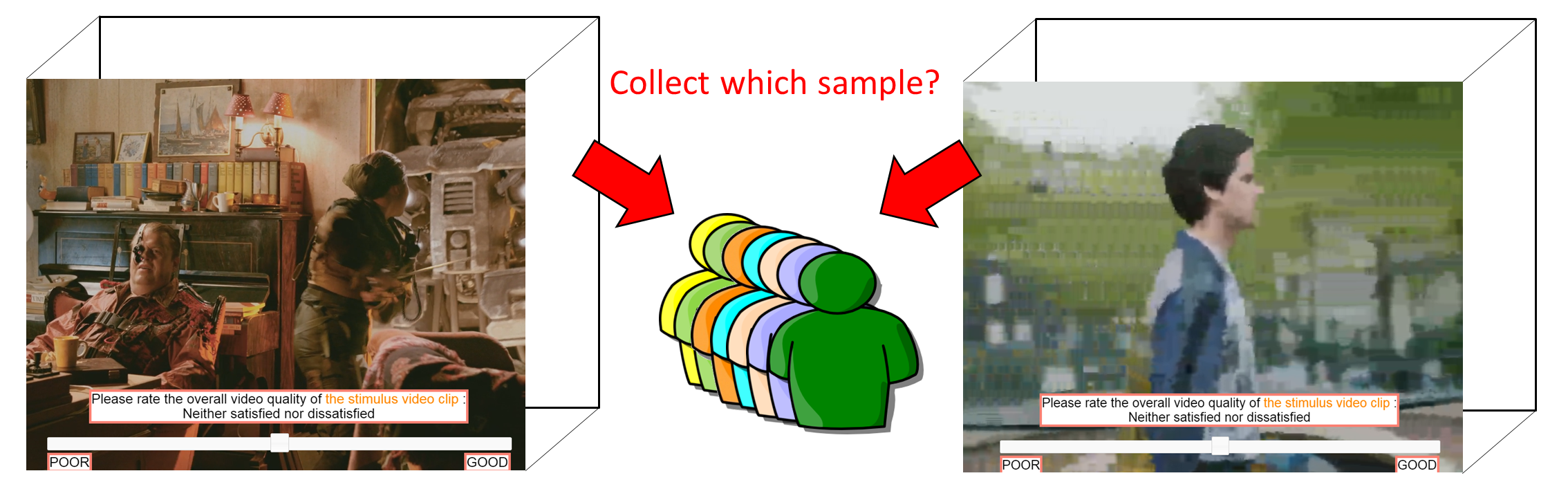
We use Bayesian learning to model the non-linear relationships between quality of experience (QoE) and multiple factors.
Our experiment shows that active sampling can be used to reduce the number of samples collected from crowdsourcing for building the model, but the users' perception of the video quality would be affected by the active learning methods (Paper).
Student Modeling and Prerequisite Verification in Knowledge Tree
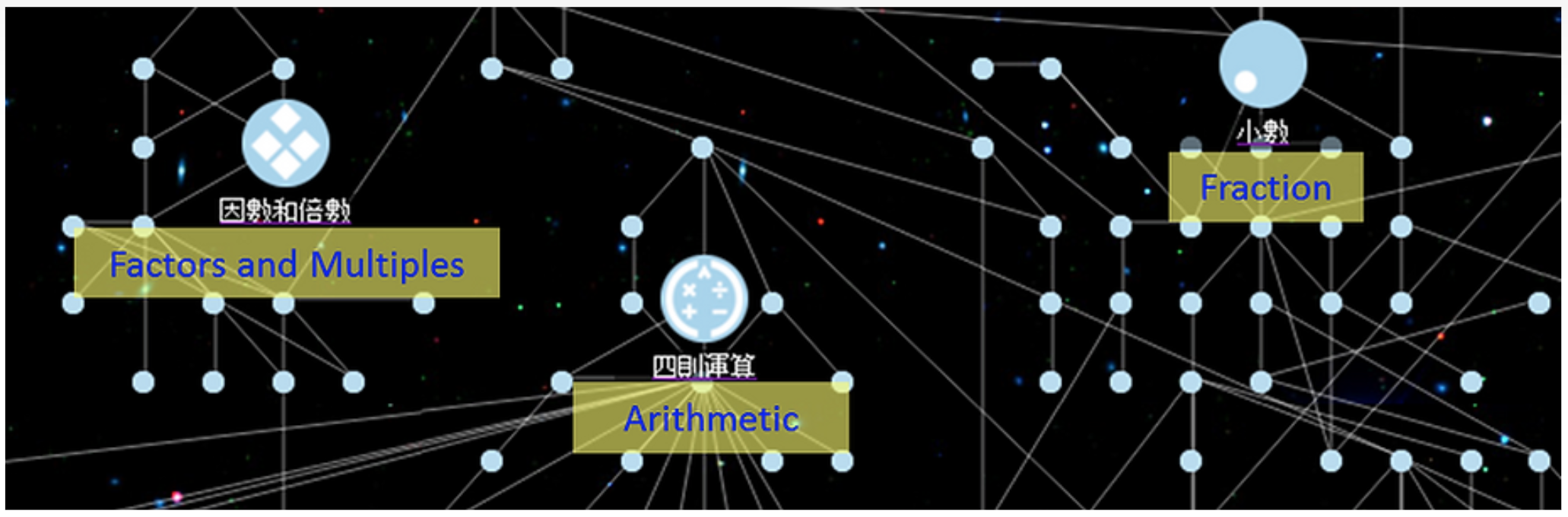
We extract answering logs of the exercises from Junyi Academy, an E-learning website similar to Khan Academy.
We use crowdsourcing and machine learning to discover prerequisite relationships between exercises. Based on that, we design a mechanism of adaptive test to improve the learning experiences of Junyi academy (Paper, Presentation, Demo, Code, Dataset).
Emphasize Uncertain Examples in Supervised Learning
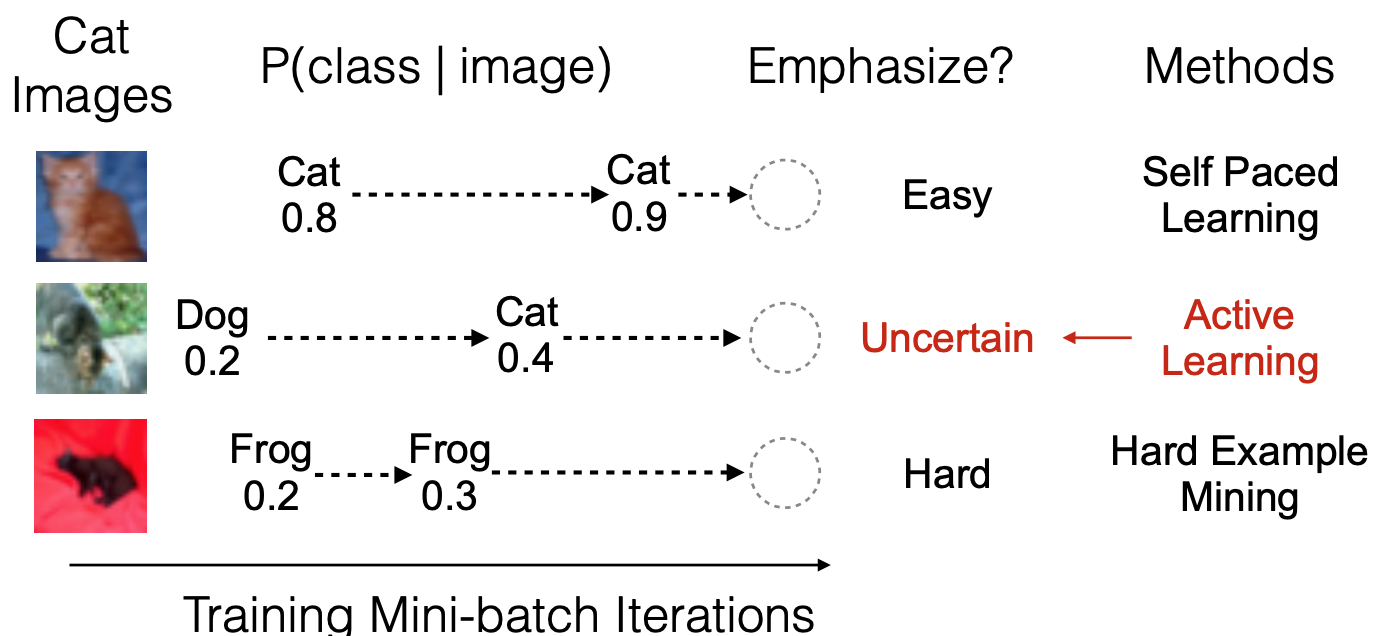
Inspired by active learning, we propose two alternatives to re-weight training samples based on lightweight estimates of sample uncertainty in stochastic gradient descent (SGD). Experimental results on six datasets show that our methods reliably improve accuracy in various network architectures, including additional gains on top of other popular training techniques (Paper, Poster).
Natural Language Processing
Distributional Inclusion Vector Embedding for Unsupevised Hypernym Detection
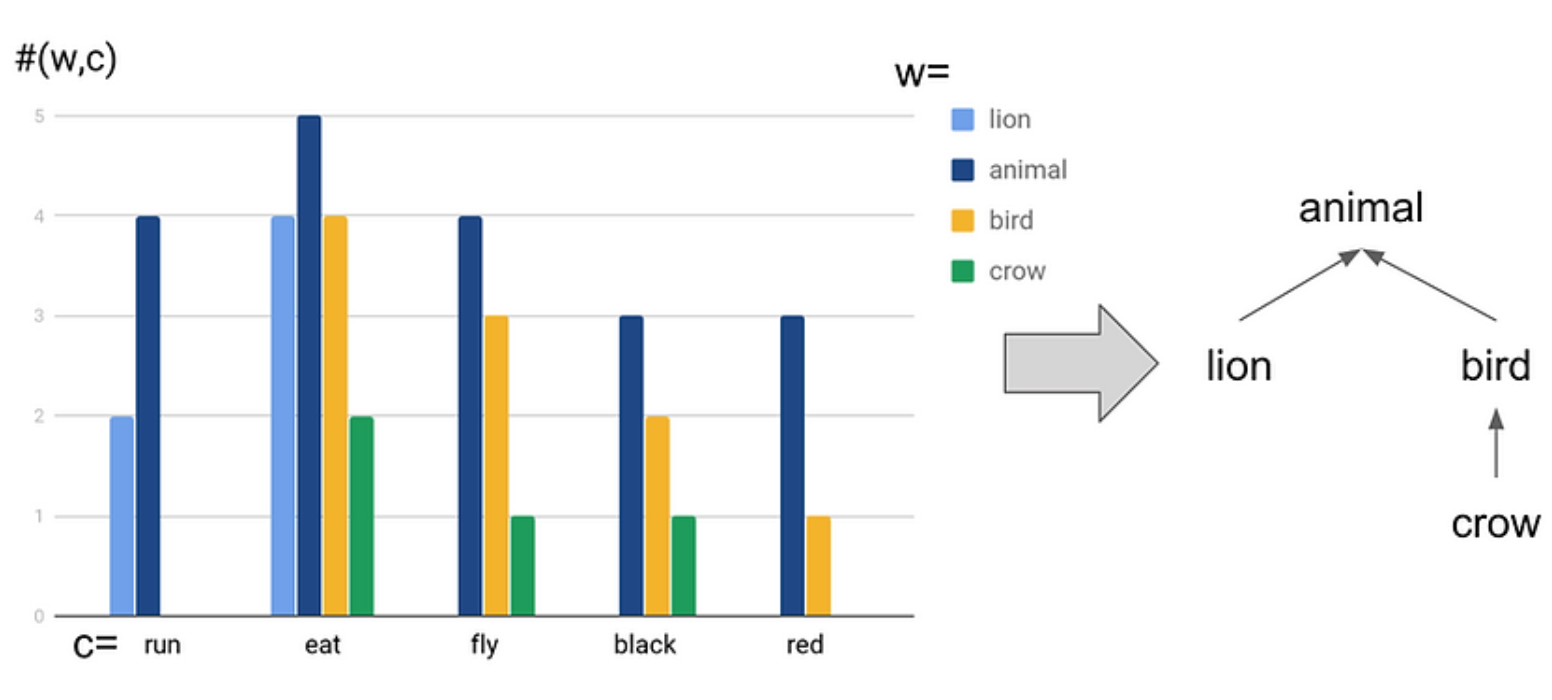
We propose a novel word embedding method that preserves the distributional inclusion property in the sparse-bag-of-word (SBOW) feature. The embedding can be used to predict the generality of words, detect the hypernym relation, and discover the topics from the raw text simultaneously. The extensive experiments show that the embedding effectively compresses the SBOW, and achieves new state-of-the-art performances on the unsupervised hypernym detection tasks (Paper, Code, Poster). We also show that DIVE could help us to do word sense induction more efficiently (Paper, Slides).
UMASS TAC-KBP 2016 System for Relation Extraction
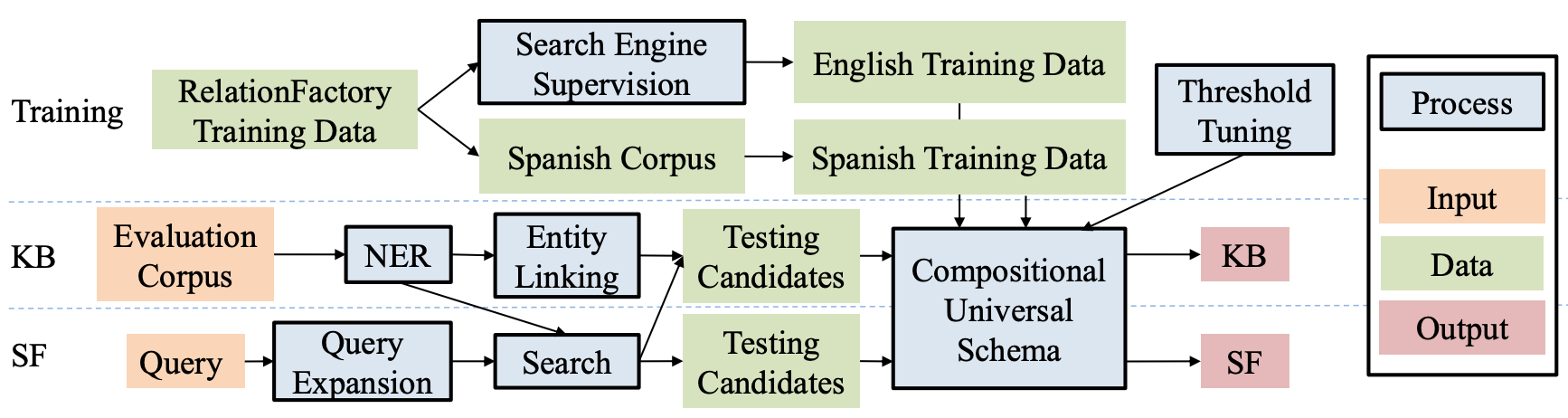
TAC-KBP is one of the most challenging text-based information retrieval tasks. We integrate research that is done in UMASS IESL in the past year, including embedding linker, multilingual Universal Schema, and LSTM sentence embedding. We perform extensive error analysis and develop some novel techniques (such as using a search engine to reduce noise in training data) to tackle the problems (Paper).
Computer Vision (Unsupervised Clustering and Matching)
Decomposition of Multiple Foreground Co-segmentation
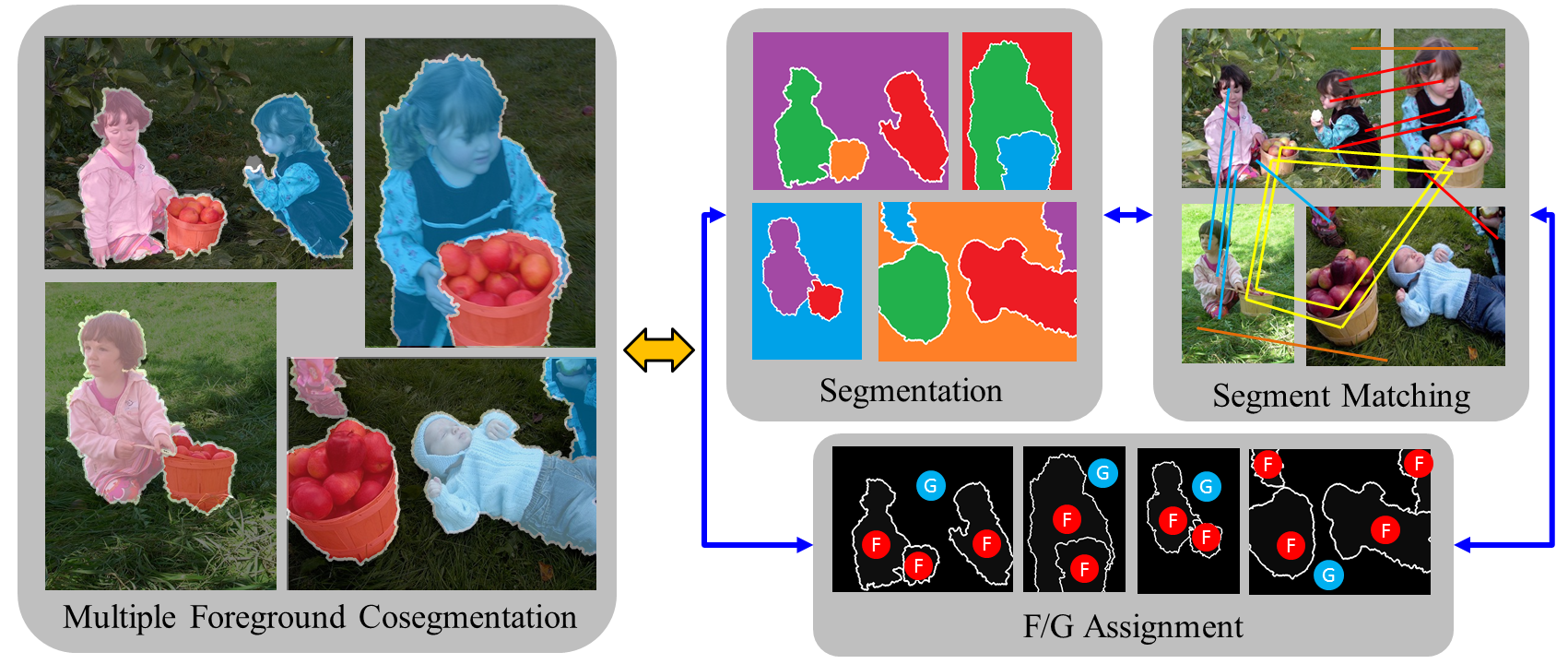
We proposed an efficient algorithm that decomposes the unsupervised Multiple Foreground Co-segmentation problem into three sub-problems: segmentation, matching, and figure-ground classification.
Our method improves the accuracy of the state-of-the-art method by 13% in a standard benchmark (Paper).
Hierarchical Image Segmentation without Training
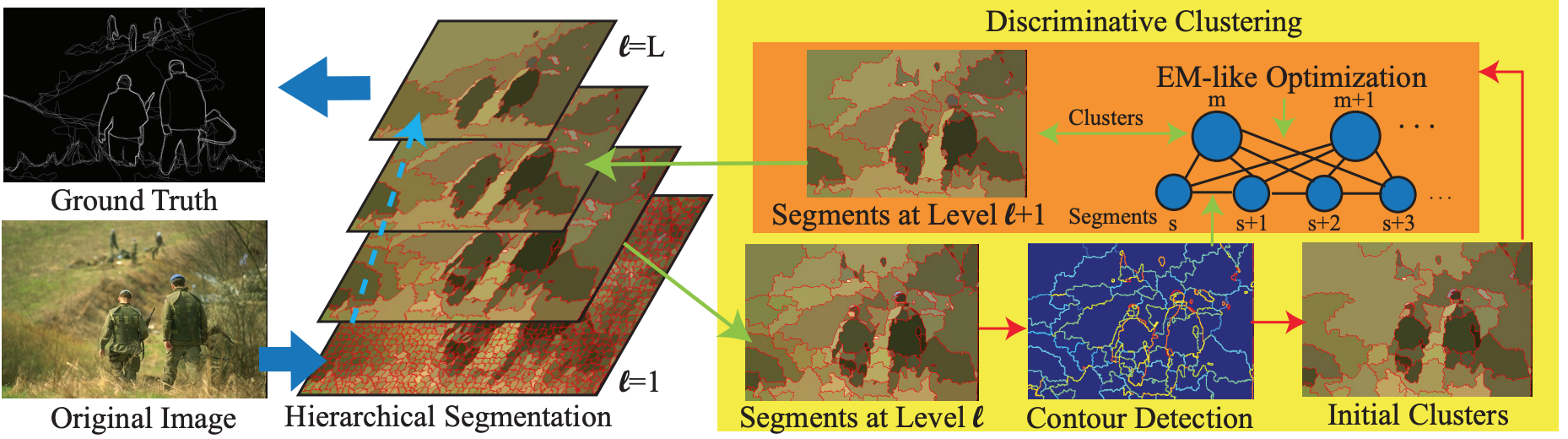
We proposed a general framework that applies classifiers with different complexity to discriminate segments in an image.
Our unsupervised hierarchical segmentation results achieve similar or better performance in several standard benchmarks compared with the current state-of-the-art methods based on supervised learning (Paper, Poster).
Superpixel-Based Large Displacement Optical Flow
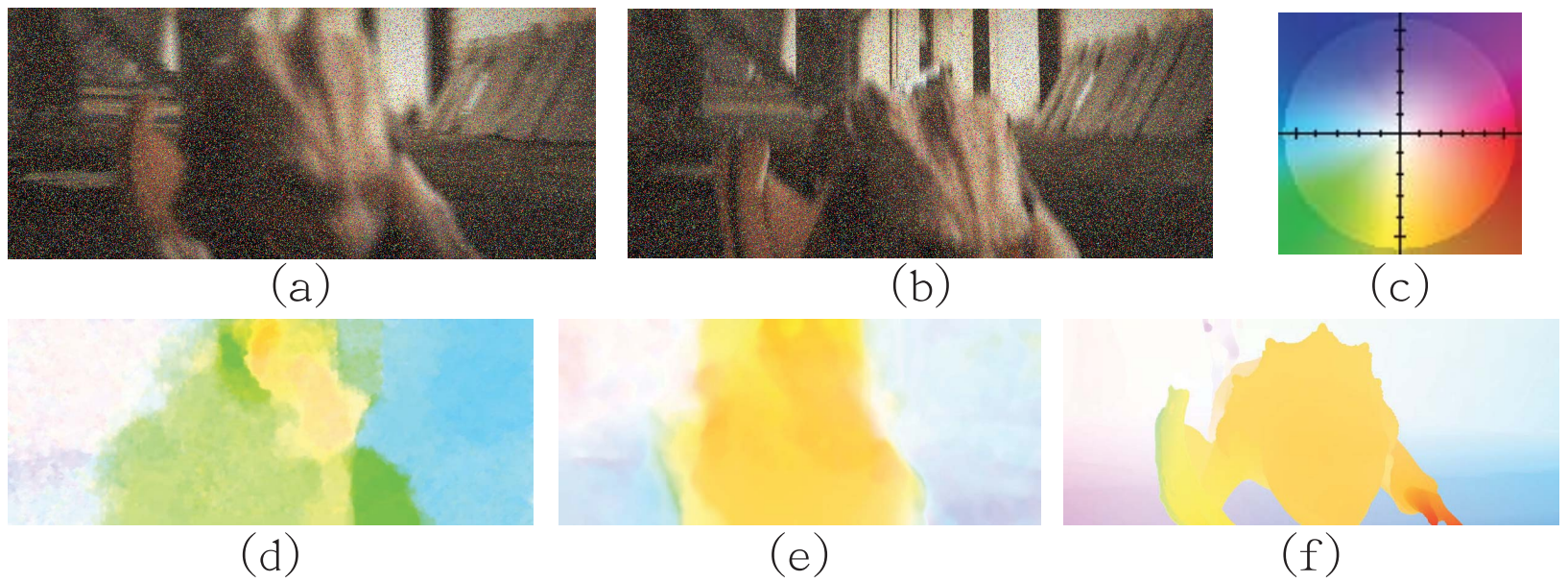